3 credits
Who is this course for?
This course is for people who want a better understanding of cause and effect, randomised experiments, do-calculus and graphical models. With knowledge of these concepts, you can answer the following questions:
- Why causal inference?
- How can causal inference improve decision making?
- What would be the potential outcome given a certain decision?
- How to represent different causal relations in terms of what causes what?
- How can machine learning methods take advantage of causal inference concepts?
What will you learn from this course?
This course aims to discuss how to extract causal information from empirical data. This course also discusses some examples that show how to use causal inference topics in machine-learning contexts.
What is the format for this course?
Instruction type: Each lecture is delivered through a video conference tool offered by a university teaching platform, followed by a practical lab assignment in Python, provided as a Jupyter notebook, which allows the participants to dig into the concepts presented in the lecture and put them to practice. Teaching is in English and online.
Frequency: This course spans nine weeks of instruction.
Examination: Exams will consist of completed lab exercises and project.
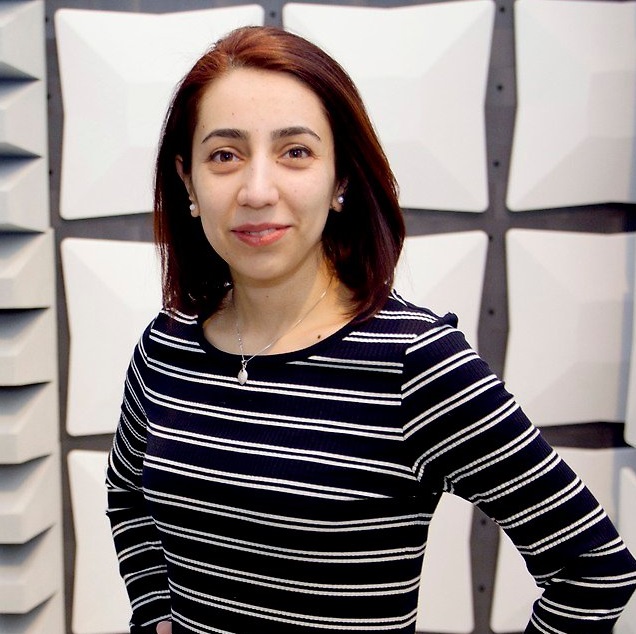
Course responsible:
Sepideh Pashami